Machine Learning Teaches Scammers a Lesson
Online deals open opportunities for theft.
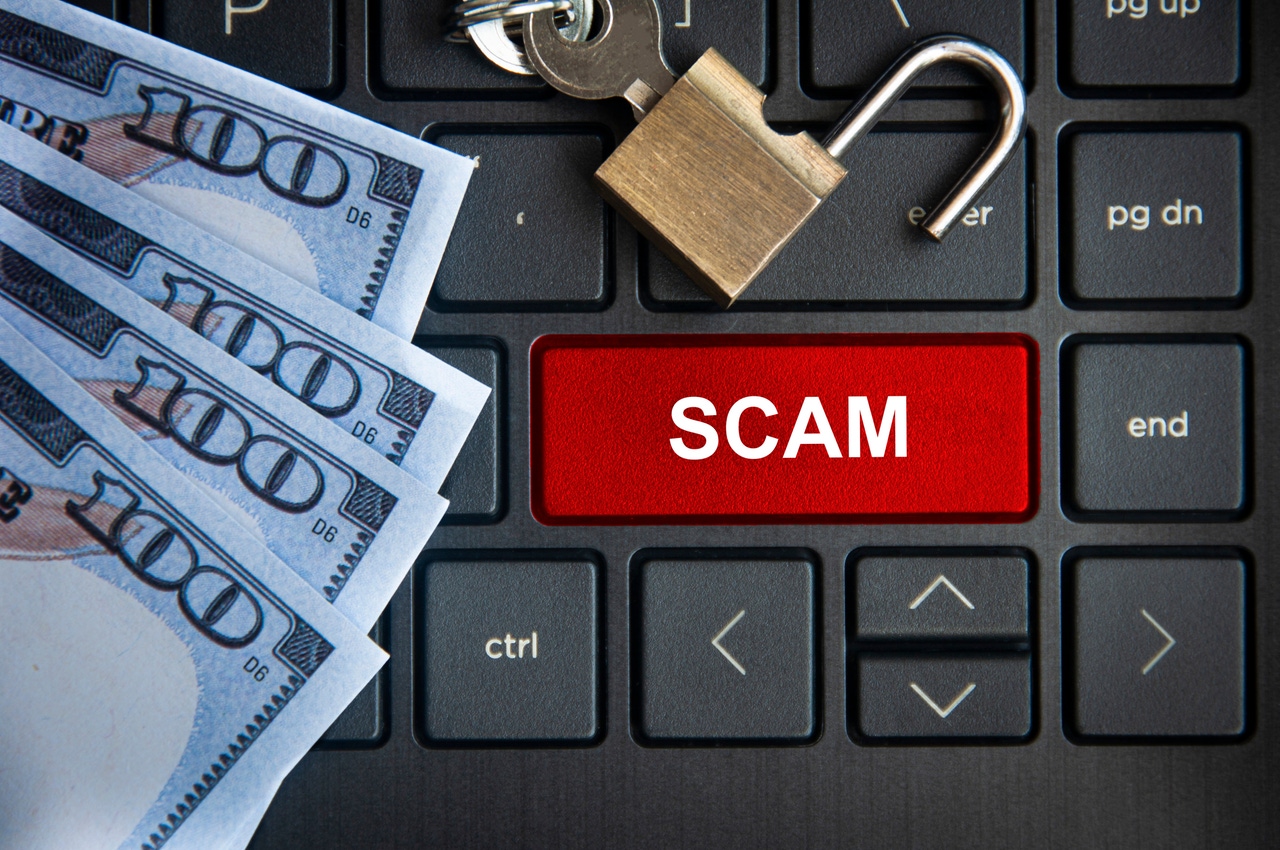
The seemingly bad news for the car dealership is that a would-be $150,000 vehicle transaction didn’t go through.
Yet, the good news is that the “deal” didn’t go through.
That’s because it was a con attempt. New anti-fraud technology red-flagged it, thwarting the perpetrator before he got away with the goods.
“The technology stopped the transaction, and on the first day of its test mode at the dealership,” James Maguire, senior director of product marketing at Experian, a data analytics and consumer reporting company, tells Wards.
Dealerships are alluring targets for scam artists looking to score big. Fraudsters sometimes do it in person, often armed with stolen identities. But the growth of digital auto retailing in recent years has also ginned up online auto retailing fraud.
“It’s challenging enough to verify the identity of someone sitting across from you at a dealership, let alone the identity of someone on the internet,” John Pappanastos, CEO of dealership finance and insurance provider EFG Companies, tells Wards.
Point Predictive, an anti-cybercrime company, analyzed millions of suspicious auto credit applications and found five top risk patterns. Those are the use of:
Social Security numbers issued after 2011 or before the borrower was born.
Fake employers or shell companies that, for a fee, pose as real employers and verify employment.
Fake employment phone numbers that are operable through the internet.
Home phone numbers that ’don’t match the identity name.
False tradelines (accounts on credit reports) and fraudulent zombie debts to boost credit scores.
Point Predictive reports that auto lenders are at high risk of synthetic identity, which blends personal information from various sources.
As such fraud increases, perpetrators new tactuseics. However, many businesses still use rules-based analytics models to identify and flag suspected fraud.
Because of the changing fraud and risk landscape, businesses instead should arm themselves with a combination of recognition and fraud-management software, says Maguire. “It’s a multi-layered approach.”
Experian says its anti-fraud services helped clients avoid $11 billion in fraud losses globally last year.
Machine learning software is a modern-day, high-tech crime stopper making the scene, although adoption rates lag somewhat.
“Machine learning identifies fraud risks early on,” Maguire says. “It’s superior to rules-based models because the technology identifies both known and unknown trends in large data sets.”
WA Podcast_13
When a new fraud trend or type emerges, machine learning can identify it and immediately flag it for further investigation, he says.Large numbers of transactions and data sets are analyzed automatically, extending fraud prevention measures. Consequently, novel and existing fraud risks are identified quickly.
But the business world in general is not immediately embracing machine learning to fight fraud, according to Experian’s 2023 Identity and Fraud Report.
Still, many companies eventually plan to add machine-learning models to their fraud-prevention tool kits.
While the cost of implementing the technology was listed as an obstacle by 25% of businesses, 35% also cited “implementation complexity” and “unclear or conflicting internal decision processes” as the greatest perceived hindrances associated with implementation.
Experian says this suggests many enterprises have their machine-learning plans on hold as they wait for more easily integrated solutions and alignments with internal priorities.
The 2023 Experian Identity and Fraud Report is partly tied to a March survey that surveyed more than 200 North American businesspeople about their fraud management and customer identification and authentication processes.
About the Author
You May Also Like